OUR LATEST ARTICLES
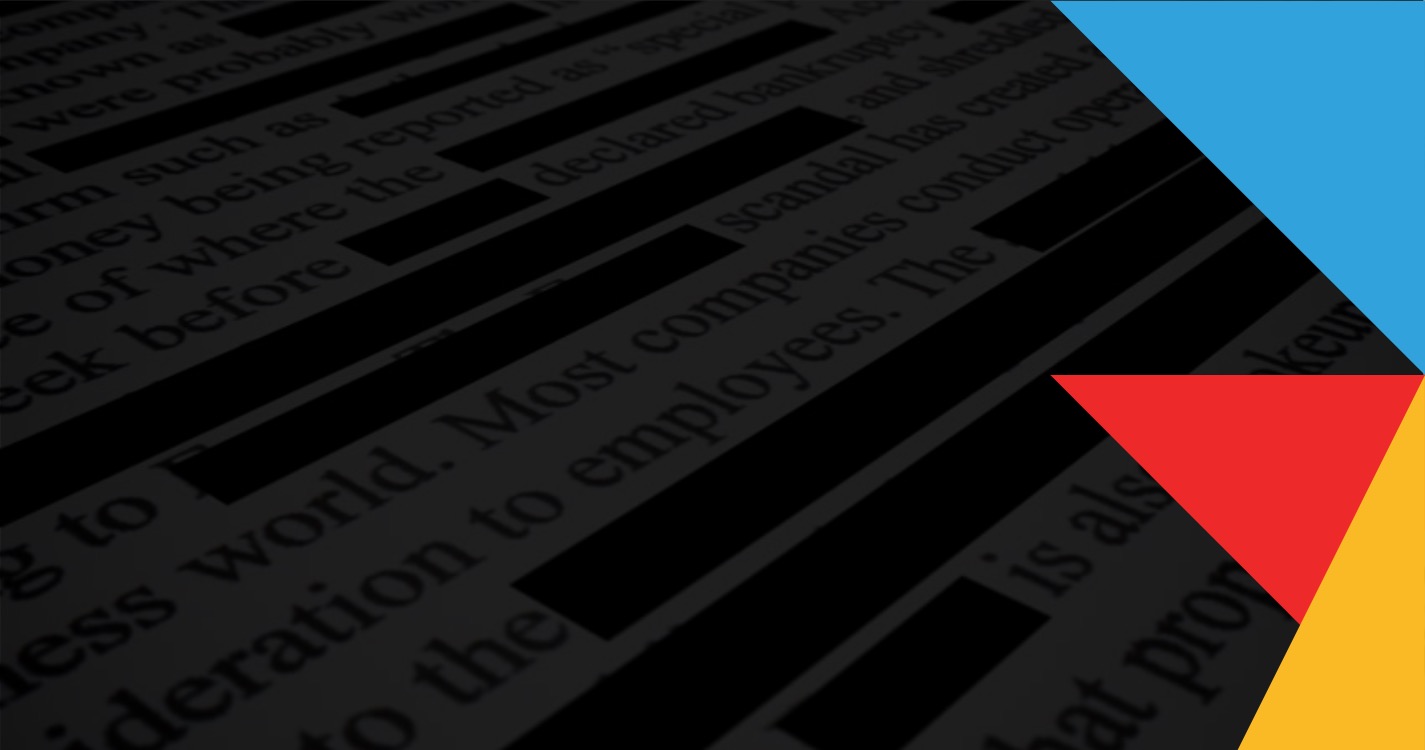
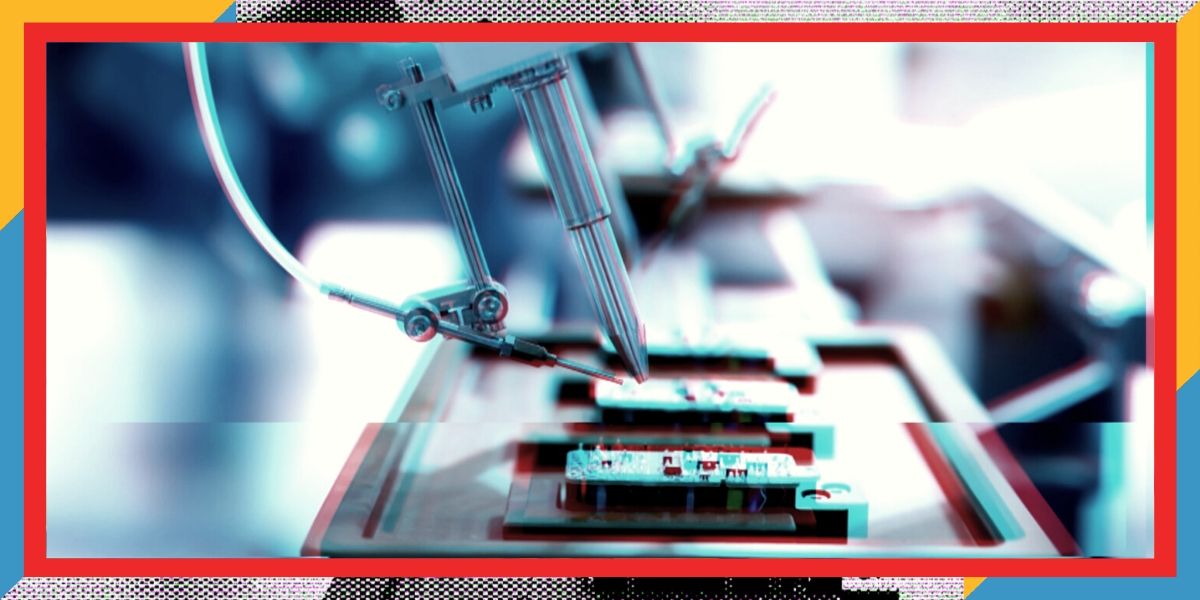
Case Study: Using Generative Design for Microprocessor Product Development
A Fortune 500 microchip design company engaged Manceps to help optimize and improve existing AI and Machine Learning solutions used in their research, design, and development process. By refining their models and developing a private ML platform architecture to support rapid prototyping and inference, the company was able to accelerate their product development cycle and streamline deployments of reliably trained, tuned and validated models at scale to their manufacturing customers.
Microchip design is exceedingly complex with several handoffs to various engineering departments along the way.
Due to the proprietary nature of their work, our client was extremely concerned about security. All datasets and Machine Learning models needed to be carefully secured. This meant designing and deploying solutions in air-gapped data centers with highly encrypted solutions.
Given the complexity of the data we were working with, Manceps needed to design a robust and reliable infrastructure from which to serve these models.
Deployed models needed to be able to deliver accurate results from new data, which could be difficult when data isn’t being processed in the cloud. Edge ML serving solutions allowed the client to continue to optimize their models without exposing the organization to additional security risks.
After a detailed design discovery effort, our team went to work on several ML-related tracks to support various stages of the client’s product development process.
The first step was to review the Machine Learning applications in the design process in order to make it easier for researchers to explore and test novel chip design ideas. We inspected their existing ML code for opportunities for improvement. We also facilitated the design review process by streamlining the ability of their design software to compute and retrieve results.
In addition to enhancing the ML models themselves, we also architected the IT infrastructure required to reliably deploy these systems. This meant redesigning their computation platform to composable, portable, highly scalable and industry-standards based clusters.
Continued research into model architectures and data-feature extraction improved performance and accuracy while providing unforeseen insights into physical modeling.At the same time, they were able to accelerate their research cycle by using an effective platform of both hardware and software.
21.11.2019
Get notified when we publish a new story.
Our Most Recent Articles
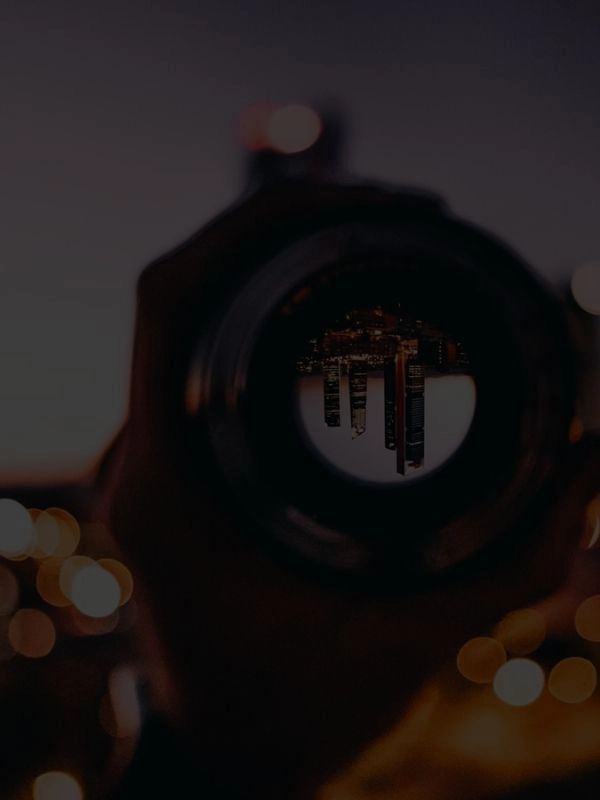